北京航空航天大学团队提出SIDM框架,利用结构信息原理优化分层强化学习,减少对先验知识的依赖
> 作者:曾祥华
分层强化学习(Hierarchical Reinforcement Learning, HRL)为解决复杂长时序任务提供了一种有前景的思路,但其策略的有效性往往依赖于先验知识和关于技能定义、任务分解的手动假设。针对这一问题,来自北京航空航天大学的研究者们提出了一种基于结构信息原理的新型分层决策框架——**SIDM (Structural Information principles-based hierarchical Decision Making)**。该框架旨在降低HRL方法对先验知识的依赖性,适用于单智能体和多智能体场景,能够通过环境抽象,自适应且动态地发现和学习分层策略。SIDM的核心思想是利用决策过程(历史状态-动作轨迹)中固有的结构信息。相关研究成果以「Hierarchical Decision Making Based on Structural Information Principles」为题,已发表于**Journal of Machine Learning Research (JMLR)**。
论文链接:**[https://arxiv.org/abs/2404.09760v1]
代码链接:** https://github.com/SELGroup/SIDM
## 结构信息原理:揭示层次结构的新视角
为解决HRL对先验知识的依赖问题,研究者们引入了**结构信息原理 (Structural Information Principles)**。该理论最初被提出用于量化复杂网络(无向图)中的动态不确定性。通过最小化图的结构熵,可以自动识别出节点的分层社团结构(即编码树, Encoding Tree),其中连接紧密的节点倾向于被划分到同一个社团内。
SIDM框架的核心创新在于,将结构信息原理的应用范围从静态图结构扩展到强化学习的动态决策过程。通过分析智能体与环境交互产生的历史状态-动作轨迹,挖掘其中蕴含的结构信息,从而自适应地发现并学习分层决策策略。
---
## SIDM框架详解:三大核心组件
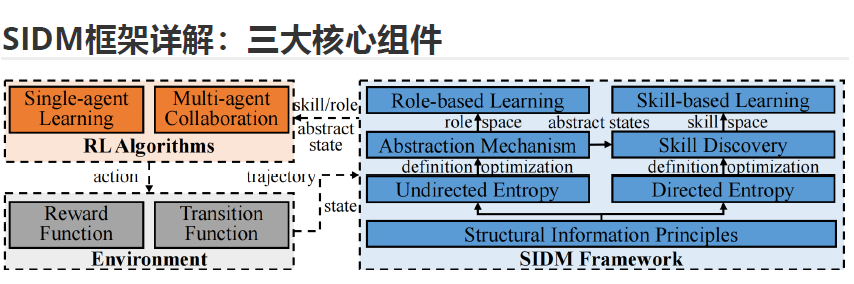
图1:SIDM框架示意图
SIDM框架由三个关键部分组成,协同工作以实现自适应的分层决策:
**1. 自适应抽象机制 (Abstraction Mechanism):**
面对原始环境信息可能存在的高维度和噪声干扰,SIDM设计了一套自适应的状态和动作抽象机制。
* **编码与相似度计算:** 首先,采用编码器-解码器结构将高维的原始状态和动作映射到低维表示空间。然后,计算状态或动作表示之间的相似性。研究中采用了计算效率较高的皮尔逊相关系数作为相似度度量,替代了计算复杂度较高的双相似性度量(bisimulation metric)。* **图构建与稀疏化:** 基于计算出的相似度,构建一个加权的无向图(状态图或动作图),其中节点代表状态(或动作),边的权重表示相似度。为了减少图中弱连接(低相似度)边的干扰,采用了一种基于一维结构熵最小化的kNN图稀疏化方法,从而保留关键的结构信息。* **分层聚类与表示聚合:** 接着,应用结构熵优化算法,通过迭代执行“伸展 (stretch)”和“压缩 (compress)”操作来优化图的编码树结构。这个过程将相似的状态或动作自适应地划分到不同层级的社团中。最后,基于优化得到的编码树以及每个节点的结构熵权重,通过一个聚合函数计算出各社团的抽象表示(抽象状态或抽象动作)。
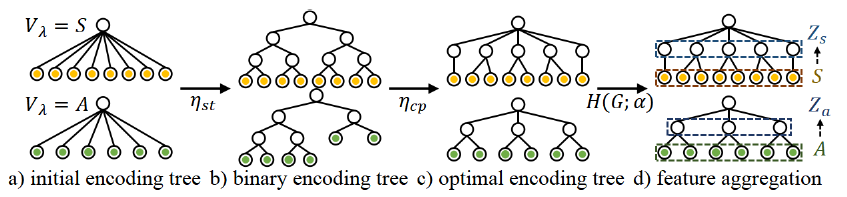
图1:抽象机制示意图
**2. 定向结构熵与技能发现 (Directed Structural Entropy & Skill Discovery):**
标准的结构信息原理主要针对无向图。然而,在强化学习中,状态之间的转移通常是有方向性的(例如,从状态A转移到状态B可能比从B转移到A更容易)。为了精确建模这种非对称性,SIDM对结构信息原理进行了扩展,提出了**定向结构熵 (Directed Structural Entropy)**。
**有向图调整:** 首先,提出了一种有向图调整算法,通过引入最小权重的边来确保图的强连通性,并对边权重进行归一化处理,使每个节点的加权出度之和为1。这样的调整保证了图上存在唯一的平稳分布,为后续定义有向结构熵奠定了基础。
**有向结构熵定义与优化:** 基于调整后有向图的平稳分布,重新定义了节点体积和边界权重,进而给出了有向图结构熵的计算公式。然后,借鉴无向图优化的思想,利用“合并 (merge)”和“组合 (combine)”操作,设计了相应的有向结构熵优化算法,用于寻找最优的有向编码树结构 Math input errorMath input errorMath input errorMath input errorMath input errorMath input errorMath input errorMath input error$Math input error$。
**分层技能发现:** 在抽象状态空间上构建加权有向转移图 Math input errorMath input errorMath input errorMath input errorMath input errorMath input errorMath input errorMath input error$Math input error$。应用上述有向结构熵优化方法得到最优编码树 Math input errorMath input errorMath input errorMath input errorMath input errorMath input errorMath input errorMath input error$Math input error$。基于这棵编码树的不同层级 Math input errorMath input errorMath input errorMath input errorMath input errorMath input errorMath input errorMath input error$Math input error$,可以定义和发现一系列具有不同时间尺度和目标的技能(Options),记为 Math input errorMath input errorMath input errorMath input errorMath input errorMath input errorMath input errorMath input error$Math input error$。技能策略的学习受到一个基于平稳分布定义的内在奖励函数 Math input errorMath input errorMath input errorMath input errorMath input errorMath input errorMath input errorMath input error$Math input error$的引导。理论分析证明,在最高层级 Math input errorMath input errorMath input errorMath input errorMath input errorMath input errorMath input errorMath input error$Math input error$ 发现的技能与基于图拉普拉斯矩阵特征向量定义的特征选项(Eigenoption)是等价的。
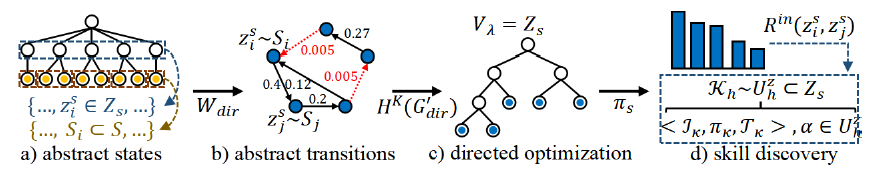
图2:技能发现示意图
**3. 分层学习方法 (Hierarchical Learning Methods):**
基于上述抽象机制和技能发现过程,SIDM框架为单智能体和多智能体场景分别设计了相应的分层学习方法:
**基于技能的学习 (Skill-based Learning, SISL - 单智能体):** 构建了一个双层HRL框架。其中,高层策略 Math input errorMath input errorMath input errorMath input errorMath input errorMath input errorMath input errorMath input error$Math input error$ 根据当前的抽象状态 Math input errorMath input errorMath input errorMath input errorMath input errorMath input errorMath input errorMath input error$Math input error$ 从发现的技能集合 Math input errorMath input errorMath input errorMath input errorMath input errorMath input errorMath input errorMath input error$Math input error$(通常包含层级K和K-1的技能)中选择一个技能 Math input errorMath input errorMath input errorMath input errorMath input errorMath input errorMath input errorMath input error$Math input error$。低层策略 Math input errorMath input errorMath input errorMath input errorMath input errorMath input errorMath input errorMath input error$Math input error$ 则负责在该技能对应的抽象状态子空间 Math input errorMath input errorMath input errorMath input errorMath input errorMath input errorMath input errorMath input error$Math input error$ 内,根据当前状态选择并执行原始动作 Math input errorMath input errorMath input errorMath input errorMath input errorMath input errorMath input errorMath input error$Math input error$,直到该技能达到终止条件。这个框架可以灵活地集成各种标准的RL算法(例如SAC)作为底层策略优化器。
**基于角色的学习 (Role-based Learning, SIRD - 多智能体):** 在多智能体协作场景下,将通过动作抽象得到的抽象动作集合 Math input errorMath input errorMath input errorMath input errorMath input errorMath input errorMath input errorMath input error$Math input error$ 定义为角色空间 Math input errorMath input errorMath input errorMath input errorMath input errorMath input errorMath input errorMath input error$Math input error$。每个角色 Math input errorMath input errorMath input errorMath input errorMath input errorMath input errorMath input errorMath input error$Math input error$ 对应于一个原始动作的子集 Math input errorMath input errorMath input errorMath input errorMath input errorMath input errorMath input errorMath input error$Math input error$。同样采用双层结构:高层策略 Math input errorMath input errorMath input errorMath input errorMath input errorMath input errorMath input errorMath input error$Math input error$ 为每个智能体 Math input errorMath input errorMath input errorMath input errorMath input errorMath input errorMath input errorMath input error$Math input error$ 分配一个角色 Math input errorMath input errorMath input errorMath input errorMath input errorMath input errorMath input errorMath input error$Math input error$。低层策略 Math input errorMath input errorMath input errorMath input errorMath input errorMath input errorMath input errorMath input error$Math input error$ 则基于全局状态 Math input errorMath input errorMath input errorMath input errorMath input errorMath input errorMath input errorMath input error$Math input error$ 在该角色允许的动作子空间 Math input errorMath input errorMath input errorMath input errorMath input errorMath input errorMath input errorMath input error$Math input error$ 内选择动作。扮演相同角色的智能体可以共享策略网络参数,从而提高学习效率和协作能力。此框架也可与多种MARL算法(如QMIX, QPLEX)结合使用。
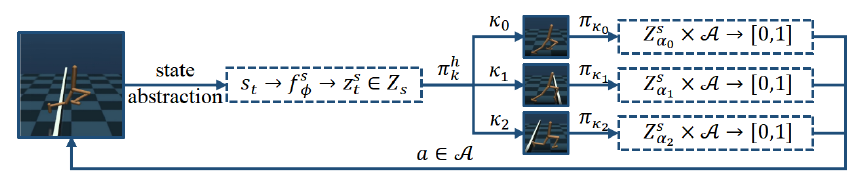
图3:单智能体技能学习示意图

图4:多智能体角色学习示意图
## SIDM 实验验证:性能提升显著,效率与稳定性俱佳
为了验证SIDM框架的有效性,研究团队在多个具有挑战性的基准环境中进行了广泛的实验评估,涵盖了从单智能体导航、连续控制到多智能体协作等多种场景。评估环境包括视觉Gridworld、DeepMind Control Suite (DMControl)、MuJoCo机器人控制以及星际争霸II微操(SMAC)套件。
**实验结果一致表明,SIDM框架及其具体实现(SISA, SISL, SIRD)相较于当前最优的基线方法,在多个方面均表现出显著优势:**
**状态抽象 (SISA):** 无论是在离线的Gridworld导航任务还是在线的DMControl连续控制任务中,SISA都展示了优越的性能。在DMControl任务上,相比基线,SISA实现的平均回合奖励最高提升了 **25.02%**(例如在hopper-hop任务中),达到目标奖励所需的训练步数最多减少了 **64.86%**(例如在hopper-stand任务中),显示出更高的样本效率。同时,SISA在多数任务中表现出更低的标准差,具有更好的稳定性。这归功于其状态抽象机制能够在压缩冗余信息和保留关键决策特征之间取得良好的平衡。
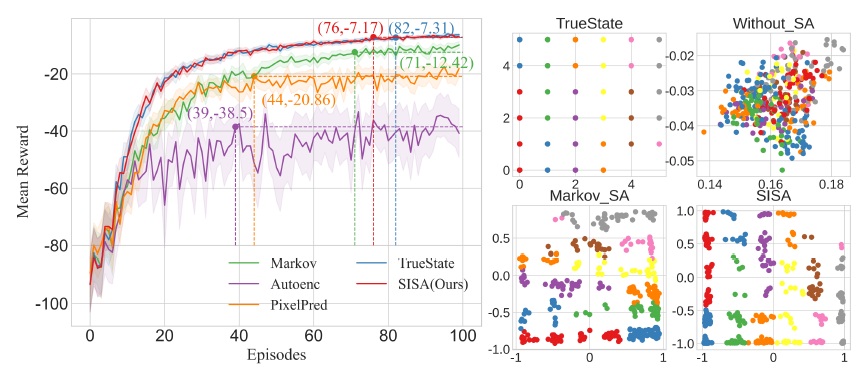
图5:6×6 Gridworld 环境导航任务在平均奖励(左)以及二维抽象状态表示的可视化(右)
**基于技能的学习 (SISL):** 在MuJoCo机器人控制任务中,SISL同样表现突出。对于双足机器人控制任务,平均奖励最高提升 **18.75%**(Hurdles任务)。在7自由度机械臂抓取任务中,平均奖励最高提升 **32.70%**(Slippery Push任务)。值得注意的是,与同样利用抽象状态发现技能的基线方法DSAA相比,SISL的平均奖励提升了约 **22.72%**;与基于多层级技能发现的基线方法Louvain相比,SISL的最终奖励标准差平均降低了 **68.83%**,显示出更好的学习稳定性。这些对比结果进一步验证了SIDM框架通过结构信息原理指导自适应分层技能构建的有效性。
**基于角色的学习 (SIRD):** 在复杂且极具挑战性的SMAC多智能体协作任务中,SIRD的表现优于所有参与对比的基线算法。尤其是在困难(hard)和超困难(super-hard)地图上,其优势更加明显。平均胜率最高提升 **5.19%**,而标准差最大降低了 **88.26%**,体现了其在学习效果和稳定性上的双重提升。综合所有14张SMAC地图来看,SIRD的最终平均测试胜率达到了 **96.7%**,明显高于次优算法ACE(92.73%)和第三优算法QPLEX(90.99%)。这表明基于结构信息原理的自适应角色发现机制对于促进复杂的多智能体协作是行之有效的。
**通用性验证:** SIDM被设计为一个通用的上层框架,能够与多种底层RL和MARL算法结合。实验验证了这一点:将SISL分别与SAC和PPO算法集成(形成SL-SAC和SL-PPO),以及将SIRD分别与QMIX和QPLEX算法集成(形成SI-QMIX和SI-QPLEX),相比原始的SAC, PPO, QMIX, QPLEX算法,集成后的版本在相应的基准测试中均表现出更优的性能或更高的学习效率。这证明了SIDM所提供的发现和利用问题内在分层结构的能力具有良好的通用性。 此外,**消融研究**也进一步证实了SIDM框架中状态抽象、有向熵优化、图构建以及边过滤等关键设计模块对于提升整体性能的积极作用。
## 总结与展望
SIDM框架提出了一种新颖的、基于结构信息原理的分层强化学习方法,其核心优势在于能够**在不依赖先验知识的情况下,自适应地发现环境或任务的内在层次结构**。通过设计的状态/动作抽象机制处理高维噪声输入,利用创新的有向结构熵概念捕捉状态转移的动态特性并发现分层的技能或角色,SIDM在单智能体和多智能体决策任务中均实现了优于现有先进方法的性能,并在学习效率和稳定性方面展现了良好的特性。 这项工作为从智能体与环境的交互数据中挖掘和利用结构信息提供了一个统一且有效的视角,有望促进分层强化学习在更广泛、更复杂的现实场景中的应用。未来的研究方向可能包括探索使用更深层级的编码树进行状态-动作抽象,以及在更具挑战性的环境中对SIDM框架进行进一步的评估和扩展。
关于研究团队/实验室
北京航空航天大学结构信息智能研究组(SII)的研究领域瞄准结构熵理论和有原理、可解释的结构信息智能应用,包括面向现实应用场景的结构信息原理、各类监督范式表示学习、各类场景强化学习模型及其应用问题,提升新算法的精度和性能。
具体研究方向包括复杂网络数据场景结构熵理论、互联网舆情事件检测、网络水军行为对抗、多智能体&深度&分层&离线强化学习智能博弈技术,以结构熵理论为核心注重其在智能场景中可解释性和有原理的新型算法突破,提升新模型计算精度和效率优化,降低当前主流人工智能深度学习算法的黑盒影响。