Multi-agent interaction is a fundamental aspect of autonomous driving in the real world. Despite more than a decade of research and development, the problem of how to competently interact with diverse road users in diverse scenarios remains largely unsolved. Learning methods have much to offer towards solving this problem. But they require a realistic multi-agent simulator that generates diverse and competent driving interactions. To meet this need, we develop a dedicated simulation platform called SMARTS (Scalable Multi-Agent RL Training School). SMARTS supports the training, accumulation, and use of diverse behavior models of road users. These are in turn used to create increasingly more realistic and diverse interactions that enable deeper and broader research on multiagent interaction. In this paper, we describe the design goals of SMARTS, explain its basic architecture and its key features, and illustrate its use through concrete multi-agent experiments on interactive scenarios. We open-source the SMARTS platform and the associated benchmark tasks and evaluation metrics to encourage and empower research on multi-agent learning for autonomous driving.
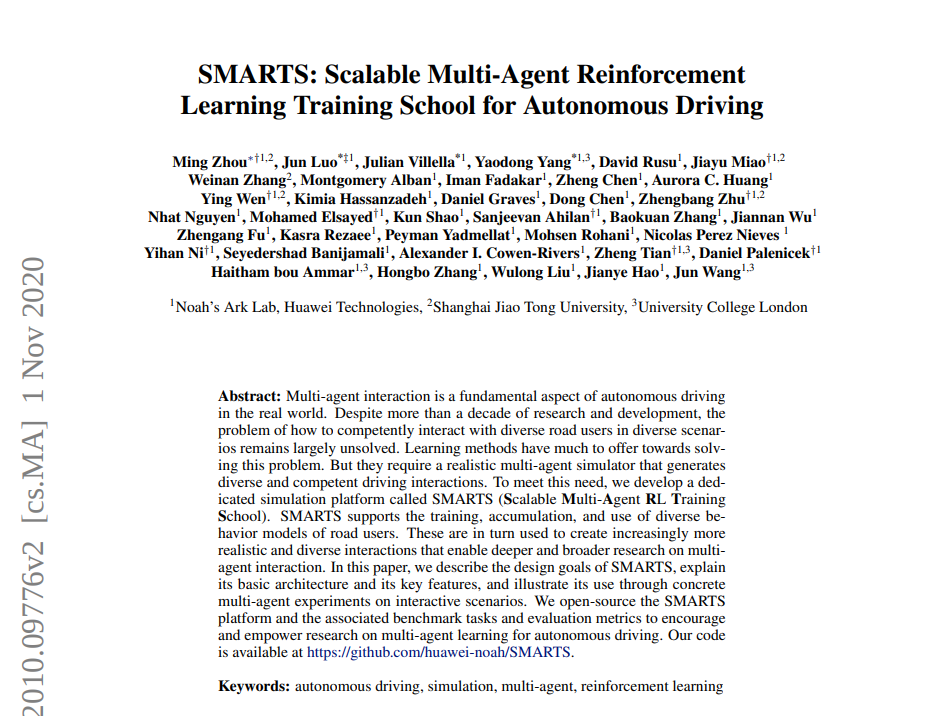
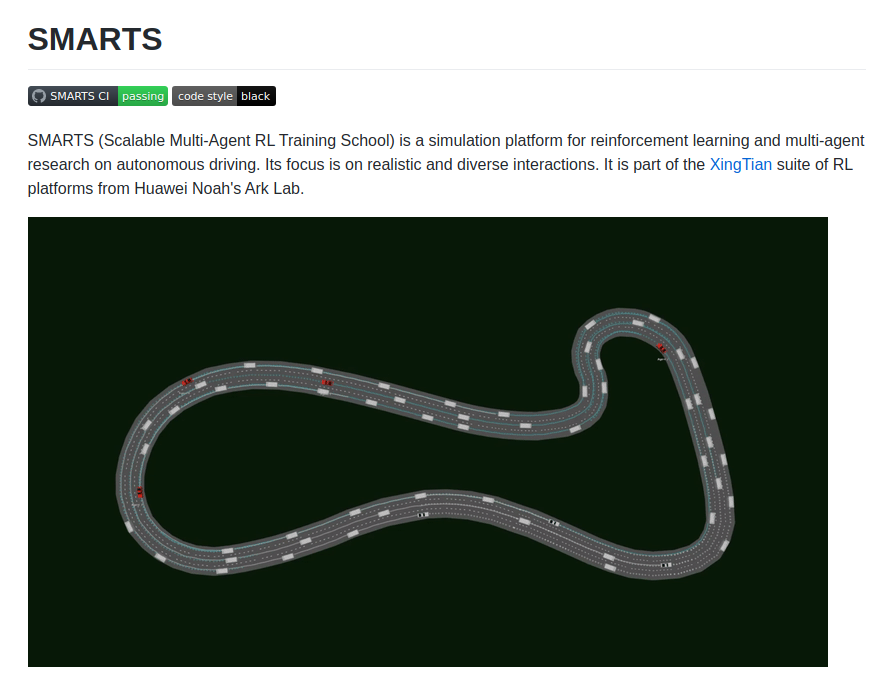
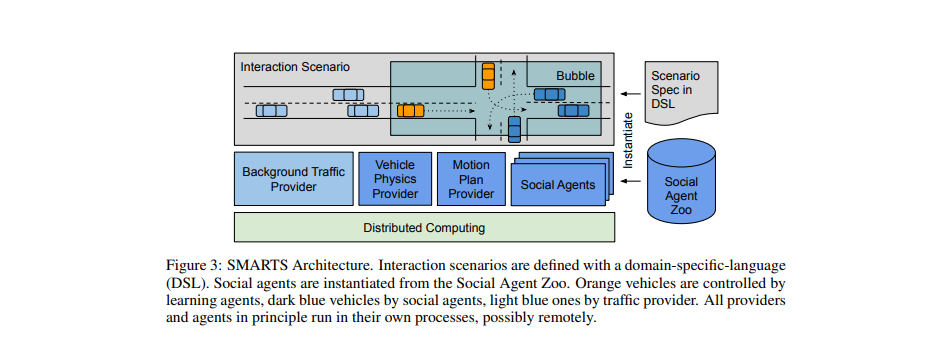
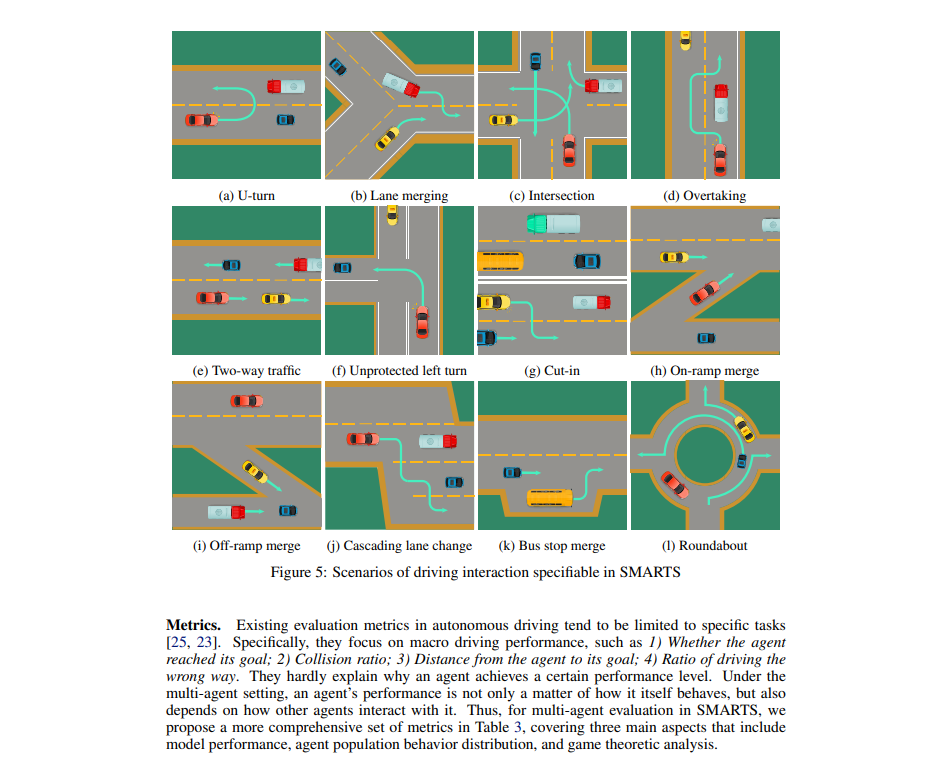
Setup
# For Mac OS X users, make sure XQuartz is pre-installed as SUMO's dependency
# git clone ...
cd <project>
# Follow the instructions given by prompt for setting up the SUMO_HOME environment variable
./install_deps.sh
# verify sumo is >= 1.5.0
# if you have issues see ./doc/SUMO_TROUBLESHOOTING.md
sumo
# setup virtual environment; presently only Python 3.7.x is officially supported
python3.7 -m venv .venv
# enter virtual environment to install all dependencies
source .venv/bin/activate
# upgrade pip, a recent version of pip is needed for the version of tensorflow we depend on
pip install --upgrade pip
# install [train] version of python package with the rllib dependencies
pip install -e .[train]
# make sure you can run tests (and verify they are passing)
make test
# then you can run a scenario, see following section for more details
Running
# build scenarios/loop
scl scenario build --clean scenarios/loop
# start supervisord
supervisord